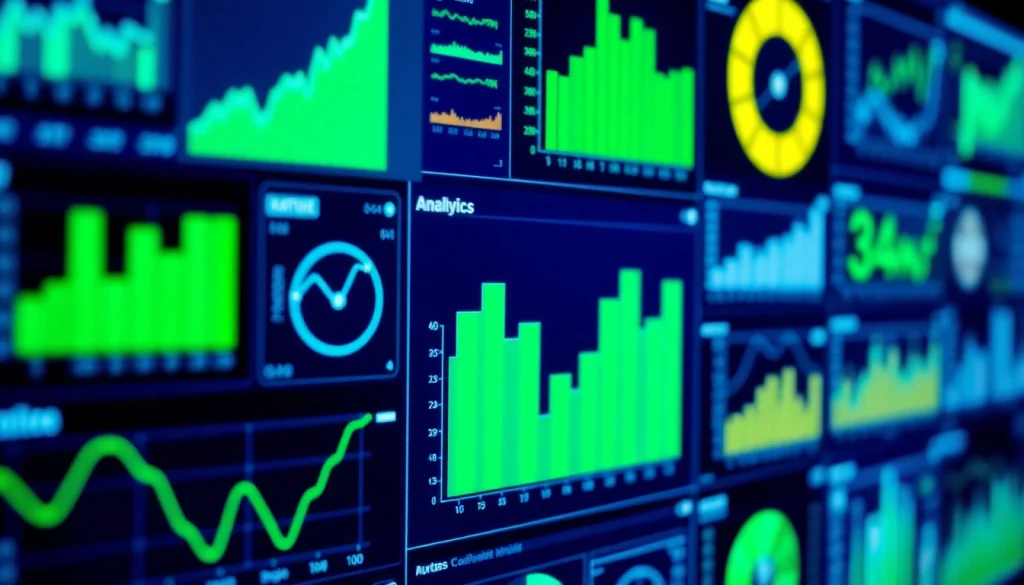
Understanding the Importance of Data Analytics
What is Data Analytics?
Data analytics is the systematic computational analysis of data. It’s a set of disciplines that uses statistical techniques, algorithms, and tools to transform raw data into meaningful information. Essentially, data analytics involves collecting, processing, and analyzing large amounts of data to identify trends, patterns, and correlations that inform decision-making processes. Different types of data analytics exist—descriptive analytics describes historical data, diagnostic analytics identifies causes of past outcomes, predictive analytics forecasts future outcomes, and prescriptive analytics recommends actions based on predicted scenarios. By harnessing these analytics, businesses can gain insight into their operations, market conditions, and customer preferences.
Benefits of Implementing Data Insights
The integration of data insights into business strategy offers numerous advantages. Firstly, it enhances decision-making capabilities; organizations backed by solid data tend to make more informed choices. Secondly, it drives operational efficiency—analyzing patterns can reveal inefficiencies, helping businesses streamline processes and reduce costs. Moreover, predictive analytics helps foresee market trends, allowing organizations to stay ahead of their competition. Implementing data analytics also improves customer experiences; businesses can tailor their services and marketing strategies according to customer behaviors gleaned from data insights. Furthermore, businesses that leverage data are more likely to innovate and adapt to changes in their industry landscape, fostering longevity and sustainability.
Challenges in Leveraging Data Effectively
Despite the considerable benefits, companies often face challenges in leveraging data effectively. Common obstacles include data quality issues—data needs to be accurate, consistent, and timely. Poor-quality data can lead to incorrect conclusions and misguided strategies. Additionally, many organizations struggle with data silos; data often sits in different departments without being shared, which limits its value. Privacy concerns and regulatory compliance also pose significant challenges, especially in industries that handle sensitive data. Lastly, a skills gap is prevalent; the shortage of skilled data analysts and data scientists can impede a company’s ability to extract actionable insights from their data pools.
Getting Started with Analytics Tools
Top Tools for Data Analysis
When embarking on a data analytics journey, selecting the right tools is crucial. Popular analytics tools include:
- Google Analytics: A powerful tool to track and report website traffic, giving insights into visitors’ behaviors.
- Tableau: This data visualization tool helps in creating interactive and shareable dashboards that present data in an accessible format.
- Power BI: A Microsoft product that allows users to visualize data and share insights across their organization.
- R and Python: Programming languages that are widely used for statistical analysis and creating data models.
- SQL: The standard language for relational database management used to manage and manipulate structured data.
Setting Up Your Analytics Environment
To effectively leverage analytics tools, a well-planned setup is vital. First, identify your key performance indicators (KPIs) that align with your business objectives. This lays the groundwork for what data to collect. Next, choose tools that fit your organizational needs—consider factors like your team’s technical skills, budget, and the scale of data you will handle. After selecting your tools, ensure that your data collection processes are in place, aligning with your KPIs. Additionally, implementing user access levels can enhance data security while allowing team members to utilize data efficiently.
Integrating Tools with https://www.informaticsview.com
Integration of analytics tools with your existing systems is crucial for seamless data flow. Start with an inventory of your current systems—understanding data sources can help choose the right analytics tools. Many platforms offer APIs for integration. Engage IT teams to assist in establishing connections and handling data migration. It’s also essential to regularly audit the integration process—ensuring data accuracy and security throughout is paramount. Moreover, training for end-users is indispensable to ensure they effectively utilize the integrated systems.
Data Insights for Decision-Making
Turning Data into Actionable Insights
Transforming raw data into actionable insights requires several steps. First and foremost, data must be cleaned and organized; this process eliminates inaccuracies and irrelevant information. Next, data analysis techniques come into play, allowing teams to dissect the data and extract meaningful patterns. Visualization tools are beneficial here, as they make the data easier to interpret. Combining statistical analysis with visualization helps stakeholders grasp key findings quickly. Lastly, organizations should have clear action plans in place—identifying how the insights can shape strategies and operational adjustments.
Case Studies: Success Stories from https://www.informaticsview.com
Several organizations across industries have harnessed data analytics successfully. For instance, a leading e-commerce platform uses customer data to analyze purchase patterns, allowing them to recommend products, thus increasing sales by 30%. Another example can be seen in healthcare, where patient data analytics have led to improved treatment plans and reduced hospital readmission rates. Industry-specific case studies highlight the benefits of tailored analytics—demonstrating how unique challenges in sectors like finance, retail, and healthcare are met with customized data strategies.
Measuring the Impact of Data-Driven Decisions
Understanding the impact of data-driven decisions is vital for organization success. To measure this impact, companies should define success metrics that align with their goals. These metrics can include revenue growth, customer satisfaction scores, or operational efficiencies. Periodic analysis should be conducted to evaluate performance against these metrics post-implementation of data insights. Additionally, businesses are encouraged to employ A/B testing—a method that compares two versions of a strategy to determine which performs better. Tracking these outcomes will illustrate the value of data-driven decision-making across business operations.
Advanced Techniques in Data Analytics
Using Predictive Analytics
Predictive analytics is instrumental in preempting future trends through statistical models and machine learning techniques. Organizations use it to forecast market trends, customer behavior, and potential disruptions. For example, businesses can predict when customers are likely to churn and implement retention strategies accordingly. The process involves collecting historical data, selecting the appropriate predictive model, and validating the findings to ensure accuracy. Tools like SAS and IBM Watson are valuable for companies looking to incorporate predictive analytics into their strategies.
Machine Learning in Data Insights
Machine learning (ML) enhances data analytics capabilities, enabling systems to learn from data patterns without direct programming. This technology can automatically improve the prediction accuracy over time. For instance, retail businesses utilize ML algorithms to optimize inventory management, analyzing purchase history to predict future demands. Moreover, machine learning can enhance customer segmentation efforts, tailoring marketing strategies to specific customer profiles based on behavioral data trends. Implementing machine learning requires a sound data infrastructure and a clear understanding of the algorithms best suited for the business context.
Future Trends in Analytics from https://www.informaticsview.com
The analytics landscape is dynamic, continuously evolving with technological advances. Currently, trends such as augmented analytics, which automates data preparation and enables natural language processing, are gaining momentum. Additionally, the rise of edge analytics—analyzing data near the source of data generation—presents new opportunities for real-time decision-making. The evolution of privacy regulations will also shape analytics, as businesses must navigate compliance while leveraging data’s power. Staying updated with these trends is crucial for organizations to maintain a competitive edge and fully exploit the potential of their data.
Improving Your Analytics Strategy
Best Practices for Continuous Improvement
Analytics is not a one-time effort; it requires ongoing refinement and adaptation to changes in business and technology landscapes. Establishing a culture of data-driven decision-making within the organization helps in embedding analytics into day-to-day operations. Regular training sessions can upskill team members on new tools and analytics techniques. Additionally, incorporating feedback mechanisms ensures that strategies can evolve in response to new information or changing circumstances. Continual gathering of data and insights should lead to a cycle of learning and improvement.
Feedback Loops and Data Refinement
Implementing feedback loops within analytics processes enables organizations to continuously learn from their data, refining their methods over time. This involves establishing systems that capture the outcomes of decisions made based on data insights, allowing for retrospective analysis of what worked and what didn’t. Adjusting algorithms based on performance outcomes helps in tweaking predictive models for better accuracy. Ensuring that these feedback loops are part of the organizational strategy facilitates a responsive approach and builds agility into the business framework.
Staying Ahead of Competitors with Data Insights
In today’s competitive marketplace, leveraging data insights is essential for staying ahead. Companies that prioritize data analytics can predict market shifts more adeptly and adapt their strategies accordingly. This proactive approach offers a significant competitive advantage, as businesses equipped with insightful data can innovate faster and respond to customer needs more effectively. Collaborating with analytics professionals or consulting firms can elevate a company’s analytical capabilities. Utilizing competitive intelligence in tandem with data analytics helps organizations to not only track trends but also foresee and pivot based on emerging market needs.